

This enables the merge of ParaView’s scientific visualization capabilities with the robustness of Python machine learning frameworks such as Pytorch, TensorFlow, Sci-Kit Learn, or Keras for the implementation of the model. Indeed, ParaView offers a thorough interface that allows the user to customize the pipeline with plugins which can be completely written in Python. The figure below shows an example of an inference result visualized in ParaView, where a velocity vector is associated to each cell.Ī ParaView Python plugin was developed for this project. This irregular structure is taken into account by the neural network, which uses spline-based convolutions layers described in (2), and is designed to take into account the geometric structure of the problem. It is important to note that the mesh provided to study this problem is irregular, and presents finer cells around the obstacle. A depiction of this model is shown above. This surrogate then outputs a discretized velocity vector field. It takes two parameters as input: the imposed velocity of the fluid and the time of the simulation. The deep neural network used to predict the results of these simulations was built using the Pytorch framework. Each member of the ensemble corresponds to a different imposed input velocity. An ensemble of simulations was performed by EDF’s CFD solver Code_Saturne. These developments have been validated on a use case representing Karman Vortex Street where a fluid (entering with an imposed velocity on the left of a rectangular domain) flows towards a cylindrical obstacle and a set of vortices forms behind it. On a second step, Catalyst has been used to monitor the training process of the deep surrogate model. In this work, ParaView has first been adapted to visualize the results produced by a deep neural network in real time (the surrogate model). They are consequently not useful for a variety of important applications, such as digital twins. These simulations produce large data sizes and take long computation times. EDF engineers regularly run Computational Fluid Dynamics (CFD) simulations for systems such as windmills, steam generators, or turbines. EDF Lab, which is the R&D department of one of the largest electric utility companies in Europe, is investigating the use of Deep-Learning to construct surrogate models. Thus, they are not well-suited for real-time applications. Solvers of partial differential equations, for instance those based on finite elements methods, often require long runs. ContextĪ surrogate model (of a numerical simulation) approximates a solver’s outputs with a low computational cost. Next, Catalyst has been used to monitor the training process of this model.
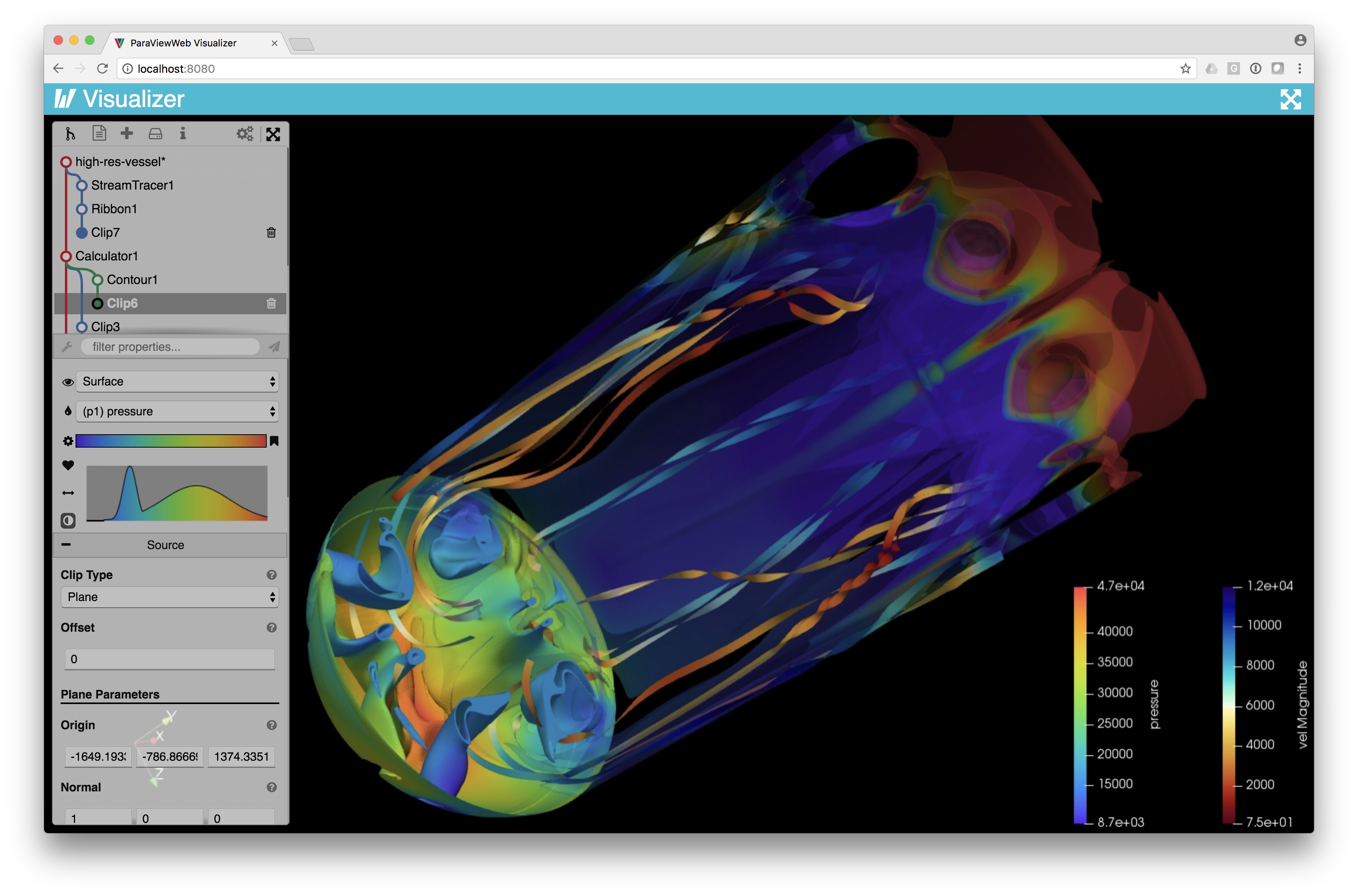
First, ParaView has been adapted to visualize the results produced by a deep-learning-based surrogate model in real time. We successfully prototyped real-time modification of the learning rate and obtained immediate feedback.This article describes two recent developments concerning the integration of Deep-learning techniques in ParaView. The steering capabilities of Catalyst 2 push this workflow forward by allowing the user to influence the learning process in real time. Using a fluid dynamics problem, the Karman Vortex Street, we can easily locate where the training succeeds and where it fails. The proposed workflow leverages the scientific visualization capabilities of ParaView to better understand the training process. Kitware partnered with EDF R&D to develop a proof of concept using the in situ capabilities of the ParaView application via the Catalyst library in order to visualize, track, and control the training of a deep learning surrogate model in real time.Ĭurrent monitoring tools lack the ability to display meaningful data in cases where standard numerical metrics are too shallow to reflect the nuances of the learning process. Surrogate models are trained to approximate the outputs of these solvers, lowering computational costs. Solvers of differential equations often require long runs, making them unsuited for time-constrained tasks.
